20 Building a Naive Bayes Classifier
- Datasets:
iris.csv
- Algorithms:
- Naive Bayes
20.1 8. Building a Naive Bayes Classifier in R
Understanding Naive Bayes was the (slightly) tricky part. Implementing it is fairly straightforward.
In R, Naive Bayes classifier is implemented in packages such as e1071
, klaR
and bnlearn
. In Python, it is implemented in scikit-learn
.
For sake of demonstration, let’s use the standard iris dataset to predict the Species of flower using 4 different features: Sepal.Length, Sepal.Width, Petal.Length, Petal.Width
# Import Data
training <- read.csv('https://raw.githubusercontent.com/selva86/datasets/master/iris_train.csv')
test <- read.csv('https://raw.githubusercontent.com/selva86/datasets/master/iris_test.csv')
The training data is now contained in training and test data in test dataframe. Lets load the klaR package and build the naive bayes model.
# Using klaR for Naive Bayes
library(klaR)
#> Loading required package: MASS
nb_mod <- NaiveBayes(Species ~ ., data=training)
pred <- predict(nb_mod, test)
Lets see the confusion matrix.
# Confusion Matrix
tab <- table(pred$class, test$Species)
caret::confusionMatrix(tab)
#> Confusion Matrix and Statistics
#>
#>
#> setosa versicolor virginica
#> setosa 15 0 0
#> versicolor 0 11 0
#> virginica 0 4 15
#>
#> Overall Statistics
#>
#> Accuracy : 0.911
#> 95% CI : (0.788, 0.975)
#> No Information Rate : 0.333
#> P-Value [Acc > NIR] : 8.47e-16
#>
#> Kappa : 0.867
#>
#> Mcnemar's Test P-Value : NA
#>
#> Statistics by Class:
#>
#> Class: setosa Class: versicolor Class: virginica
#> Sensitivity 1.000 0.733 1.000
#> Specificity 1.000 1.000 0.867
#> Pos Pred Value 1.000 1.000 0.789
#> Neg Pred Value 1.000 0.882 1.000
#> Prevalence 0.333 0.333 0.333
#> Detection Rate 0.333 0.244 0.333
#> Detection Prevalence 0.333 0.244 0.422
#> Balanced Accuracy 1.000 0.867 0.933
# Plot density of each feature using nb_mod
opar = par(mfrow=c(2, 2), mar=c(4,0,0,0))
plot(nb_mod, main="")
par(opar)
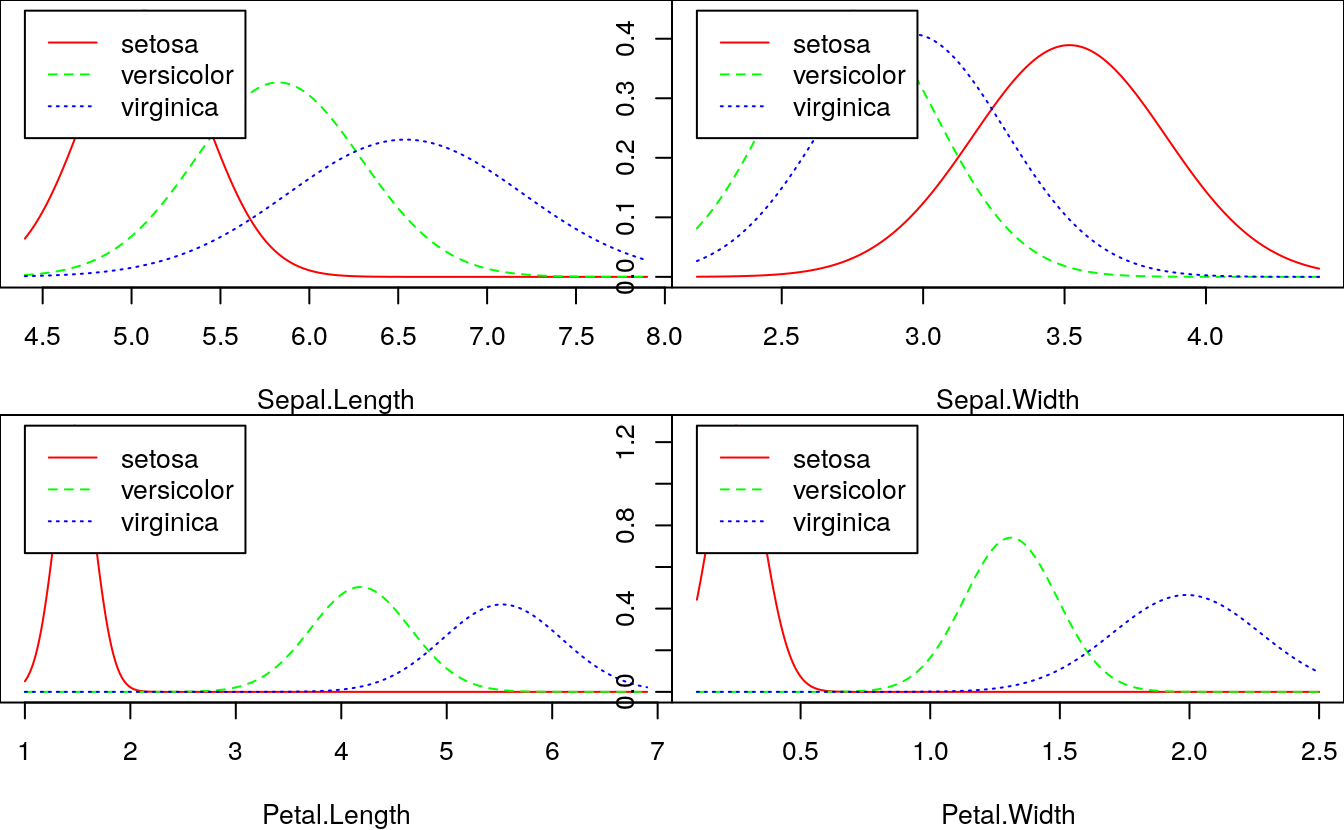
# Plot the Confusion Matrix
library(ggplot2)
test$pred <- pred$class
ggplot(test, aes(Species, pred, color = Species)) +
geom_jitter(width = 0.2, height = 0.1, size=2) +
labs(title="Confusion Matrix",
subtitle="Predicted vs. Observed from Iris dataset",
y="Predicted",
x="Truth",
caption="machinelearningplus.com")
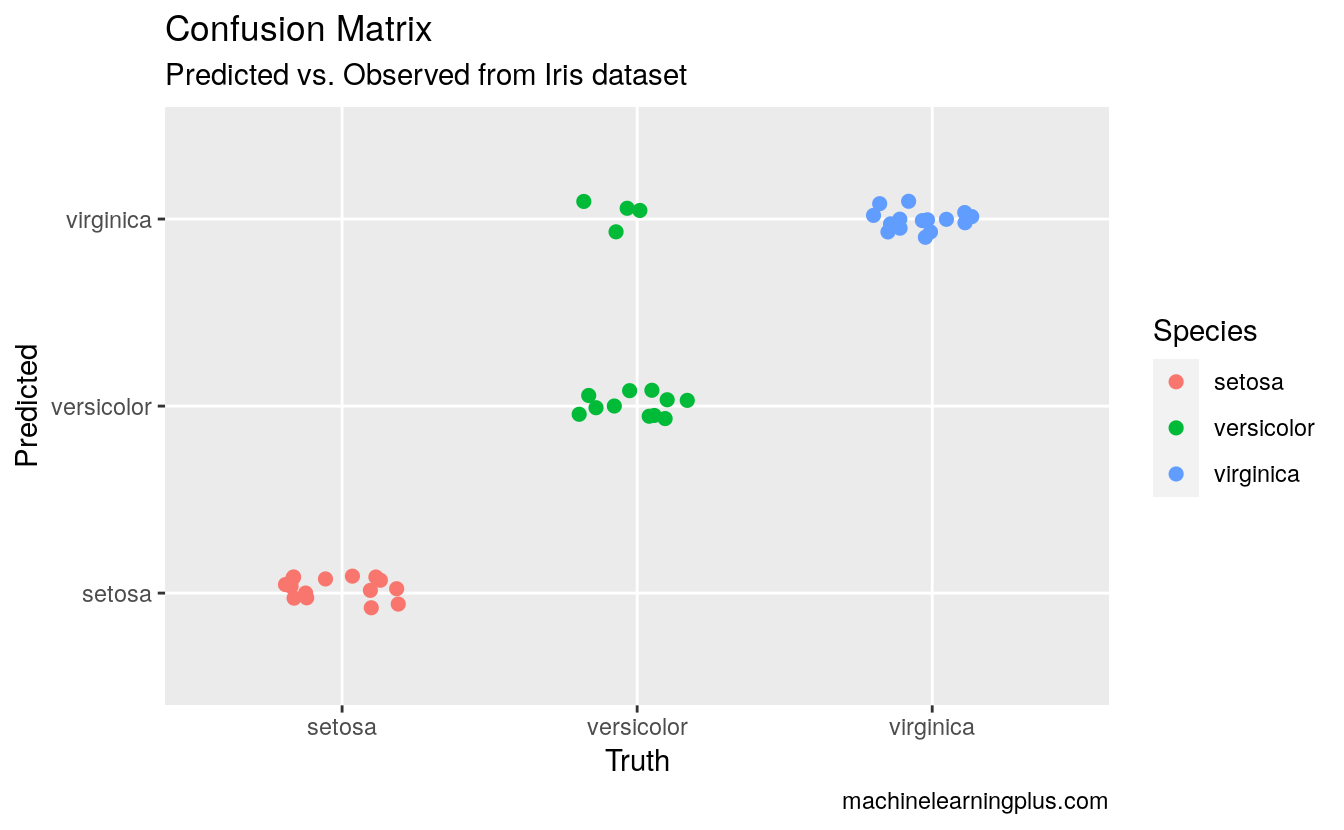