Ch. 1 Introduction
Last update: Thu Nov 19 17:20:43 2020 -0600 (49b93b1)
Remember that in the previous chapter we said that the best way of obtaining reproducible results writing Python code in Rmarkdown is creating stand-alone Python environments. The next code block is written in R and what is doing with reticulate::use_condaenv("r-python")
, is activating the Python environment r-python
to be used by Python to build the notebooks written in Rmarkdown . Later we will see how to create these environments.
R
library(reticulate)
reticulate::use_condaenv("r-python")
1.1 “Hello world”
The environment r-python
already has the basic Python libraries, among them numpy
and matplotlib
. This is one of the simplest of examples: plotting the sine of a random numpy
array.
Python
import matplotlib.pyplot as plt
import numpy as np
= np.arange(0.0, 2.0, 0.01)
t = 1 + np.sin(2*np.pi*t)
s
plt.plot(t, s)
'time (s)')
plt.xlabel('voltage (mV)')
plt.ylabel('About as simple as it gets, folks')
plt.title(True)
plt.grid("test.png")
plt.savefig( plt.show()
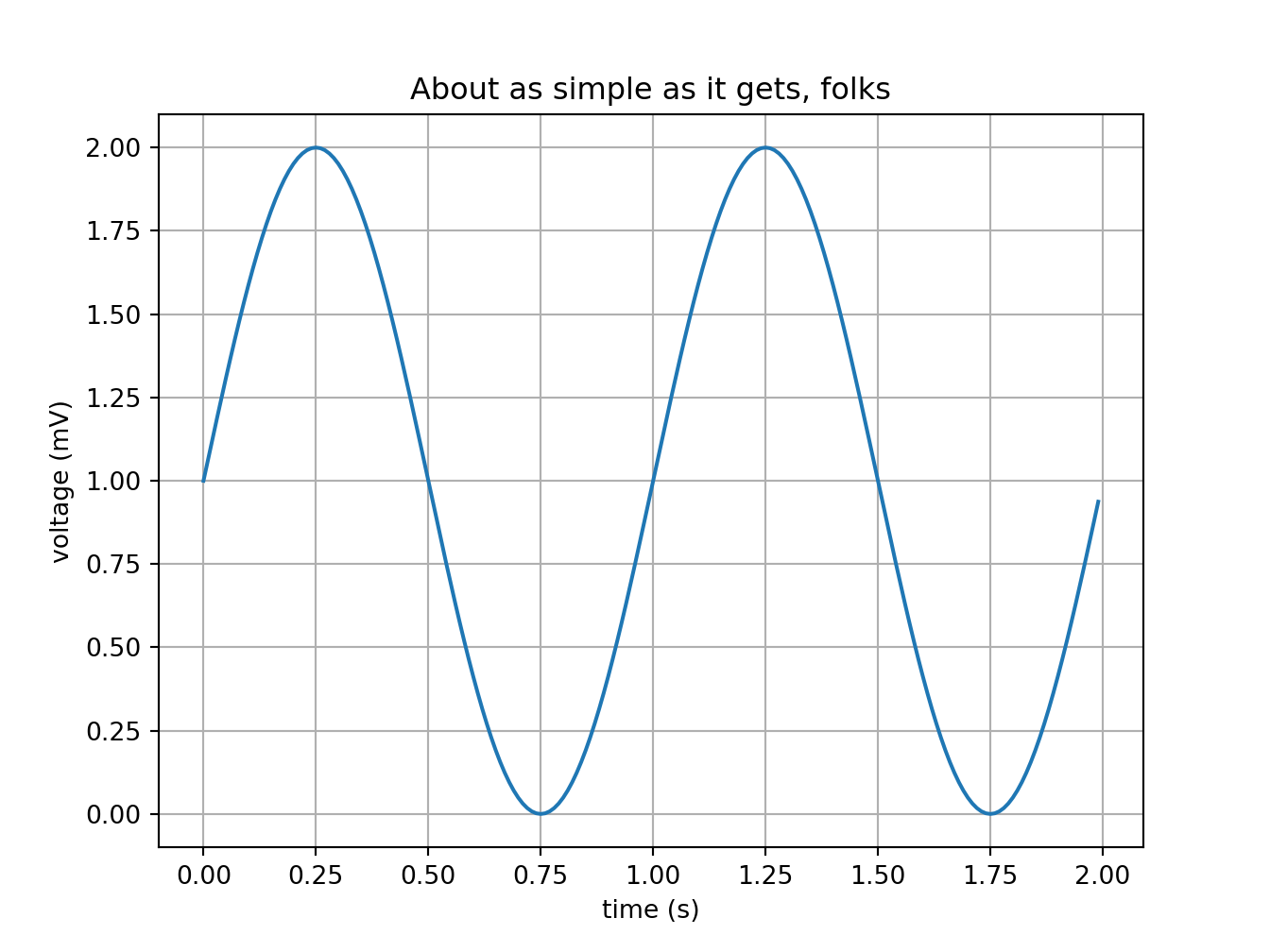
1.2 The parts of a plot
I love this plot because it helps to formulate the right question when you are looking for online assistance. Sooner of later, you will be in need to customize the \(x\) or \(y\) axis ticks in such a way that present specific data points and skip the defaults. Or, get rid of so many $x$ axis labels that are superimposing one over each other.
Python
# https://matplotlib.org/gallery/showcase/anatomy.html#sphx-glr-gallery-showcase-anatomy-py
import numpy as np
import matplotlib.pyplot as plt
from matplotlib.ticker import AutoMinorLocator, MultipleLocator, FuncFormatter
19680801)
np.random.seed(
= np.linspace(0.5, 3.5, 100)
X = 3+np.cos(X)
Y1 = 1+np.cos(1+X/0.75)/2
Y2 = np.random.uniform(Y1, Y2, len(X))
Y3
= plt.figure(figsize=(8, 8))
fig = fig.add_subplot(1, 1, 1, aspect=1)
ax
def minor_tick(x, pos):
if not x % 1.0:
return ""
return "%.2f" % x
1.000))
ax.xaxis.set_major_locator(MultipleLocator(4))
ax.xaxis.set_minor_locator(AutoMinorLocator(1.000))
ax.yaxis.set_major_locator(MultipleLocator(4))
ax.yaxis.set_minor_locator(AutoMinorLocator(
ax.xaxis.set_minor_formatter(FuncFormatter(minor_tick))
0, 4)
ax.set_xlim(#:> (0.0, 4.0)
0, 4)
ax.set_ylim(#:> (0.0, 4.0)
='major', width=1.0)
ax.tick_params(which='major', length=10)
ax.tick_params(which='minor', width=1.0, labelsize=10)
ax.tick_params(which='minor', length=5, labelsize=10, labelcolor='0.25')
ax.tick_params(which
="--", linewidth=0.5, color='.25', zorder=-10)
ax.grid(linestyle
=(0.25, 0.25, 1.00), lw=2, label="Blue signal", zorder=10)
ax.plot(X, Y1, c=(1.00, 0.25, 0.25), lw=2, label="Red signal")
ax.plot(X, Y2, c=0,
ax.plot(X, Y3, linewidth='o', markerfacecolor='w', markeredgecolor='k')
marker
"Anatomy of a figure", fontsize=20, verticalalignment='bottom')
ax.set_title("X axis label")
ax.set_xlabel("Y axis label")
ax.set_ylabel(
ax.legend()
def circle(x, y, radius=0.15):
from matplotlib.patches import Circle
from matplotlib.patheffects import withStroke
= Circle((x, y), radius, clip_on=False, zorder=10, linewidth=1,
circle ='black', facecolor=(0, 0, 0, .0125),
edgecolor=[withStroke(linewidth=5, foreground='w')])
path_effects
ax.add_artist(circle)
def text(x, y, text):
="white",
ax.text(x, y, text, backgroundcolor='center', va='top', weight='bold', color='blue')
ha
# Minor tick
0.50, -0.10)
circle(0.50, -0.32, "Minor tick label")
text(
# Major tick
-0.03, 4.00)
circle(0.03, 3.80, "Major tick")
text(
# Minor tick
0.00, 3.50)
circle(0.00, 3.30, "Minor tick")
text(
# Major tick label
-0.15, 3.00)
circle(-0.15, 2.80, "Major tick label")
text(
# X Label
1.80, -0.27)
circle(1.80, -0.45, "X axis label")
text(
# Y Label
-0.27, 1.80)
circle(-0.27, 1.6, "Y axis label")
text(
# Title
1.60, 4.13)
circle(1.60, 3.93, "Title")
text(
# Blue plot
1.75, 2.80)
circle(1.75, 2.60, "Line\n(line plot)")
text(
# Red plot
1.20, 0.60)
circle(1.20, 0.40, "Line\n(line plot)")
text(
# Scatter plot
3.20, 1.75)
circle(3.20, 1.55, "Markers\n(scatter plot)")
text(
# Grid
3.00, 3.00)
circle(3.00, 2.80, "Grid")
text(
# Legend
3.70, 3.80)
circle(3.70, 3.60, "Legend")
text(
# Axes
0.5, 0.5)
circle(0.5, 0.3, "Axes")
text(
# Figure
-0.3, 0.65)
circle(-0.3, 0.45, "Figure")
text(
= 'blue'
color 'Spines', xy=(4.0, 0.35), xycoords='data',
ax.annotate(=(3.3, 0.5), textcoords='data',
xytext='bold', color=color,
weight=dict(arrowstyle='->',
arrowprops="arc3",
connectionstyle=color))
color
'', xy=(3.15, 0.0), xycoords='data',
ax.annotate(=(3.45, 0.45), textcoords='data',
xytext='bold', color=color,
weight=dict(arrowstyle='->',
arrowprops="arc3",
connectionstyle=color))
color
4.0, -0.4, "Made with http://matplotlib.org",
ax.text(=10, ha="right", color='.5')
fontsize
plt.show()
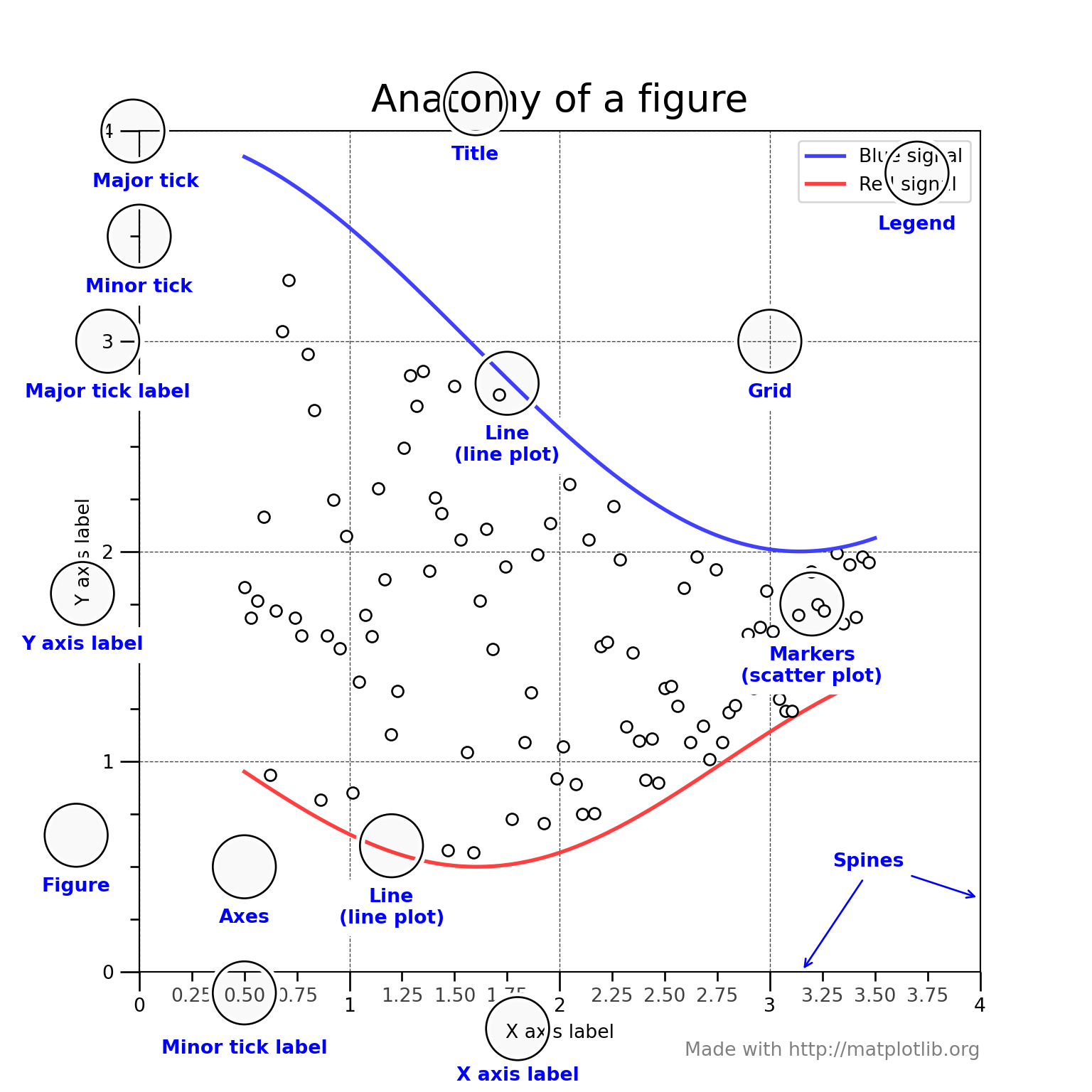
1.3 Can do business plots too …
Not precisely the kind of plots I am interested in right now, all the kinds of business plots are available in matplotlib
, including the infamous pie chart.
Python
import numpy as np
import matplotlib.pyplot as plt
'ggplot')
plt.style.use(
= plt.subplots(ncols=2, nrows=2)
fig, axes = axes.ravel()
ax1, ax2, ax3, ax4
# scatter plot (Note: `plt.scatter` doesn't use default colors)
= np.random.normal(size=(2, 200))
x, y 'o')
ax1.plot(x, y,
# sinusoidal lines with colors from default color cycle
= 2*np.pi
L = np.linspace(0, L)
x = len(plt.rcParams['axes.prop_cycle'])
ncolors = np.linspace(0, L, ncolors, endpoint=False)
shift for s in shift:
+ s), '-')
ax2.plot(x, np.sin(x 0)
ax2.margins(
# bar graphs
= np.arange(5)
x = np.random.randint(1, 25, size=(2, 5))
y1, y2 = 0.25
width
ax3.bar(x, y1, width)+ width, y2, width,
ax3.bar(x =list(plt.rcParams['axes.prop_cycle'])[2]['color'])
color+ width)
ax3.set_xticks(x 'a', 'b', 'c', 'd', 'e'])
ax3.set_xticklabels([
# circles with colors from default color cycle
for i, color in enumerate(plt.rcParams['axes.prop_cycle']):
= np.random.normal(size=2)
xy =0.3, color=color['color']))
ax4.add_patch(plt.Circle(xy, radius'equal')
ax4.axis(0)
ax4.margins( plt.show()
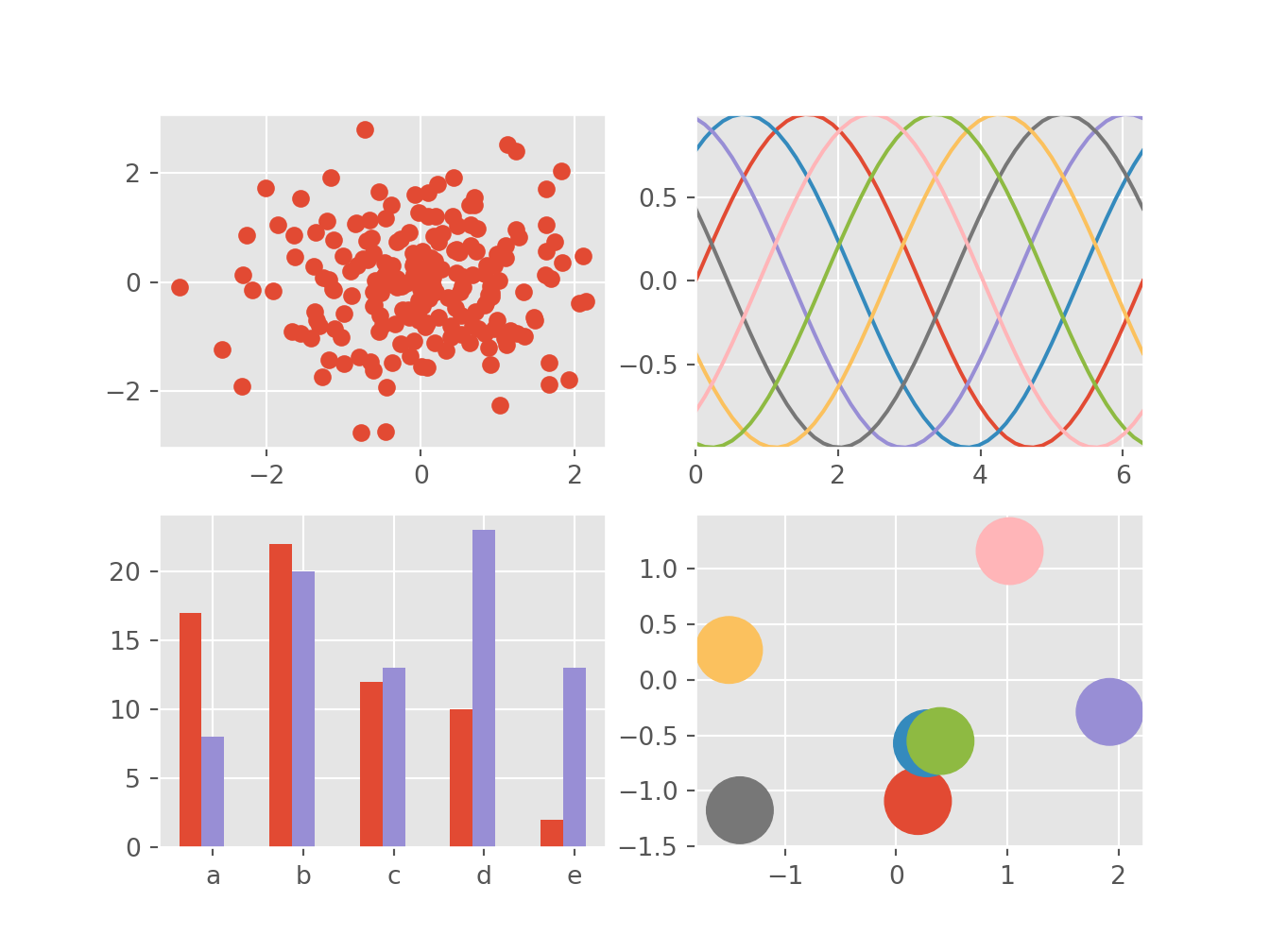
1.4 And real time …
Strip charts are the favorites for plotting real time data, from sensors, or from any other internet source.
Python
# https://matplotlib.org/gallery/lines_bars_and_markers/cohere.html#sphx-glr-gallery-lines-bars-and-markers-cohere-py
import numpy as np
import matplotlib.pyplot as plt
# Fixing random state for reproducibility
19680801)
np.random.seed(
= 0.01
dt = np.arange(0, 30, dt)
t = np.random.randn(len(t)) # white noise 1
nse1 = np.random.randn(len(t)) # white noise 2
nse2
# Two signals with a coherent part at 10Hz and a random part
= np.sin(2 * np.pi * 10 * t) + nse1
s1 = np.sin(2 * np.pi * 10 * t) + nse2
s2
= plt.subplots(2, 1)
fig, axs 0].plot(t, s1, t, s2)
axs[0].set_xlim(0, 2)
axs[0].set_xlabel('time')
axs[0].set_ylabel('s1 and s2')
axs[0].grid(True)
axs[
= axs[1].cohere(s1, s2, 256, 1. / dt)
cxy, f 1].set_ylabel('coherence')
axs[
fig.tight_layout() plt.show()
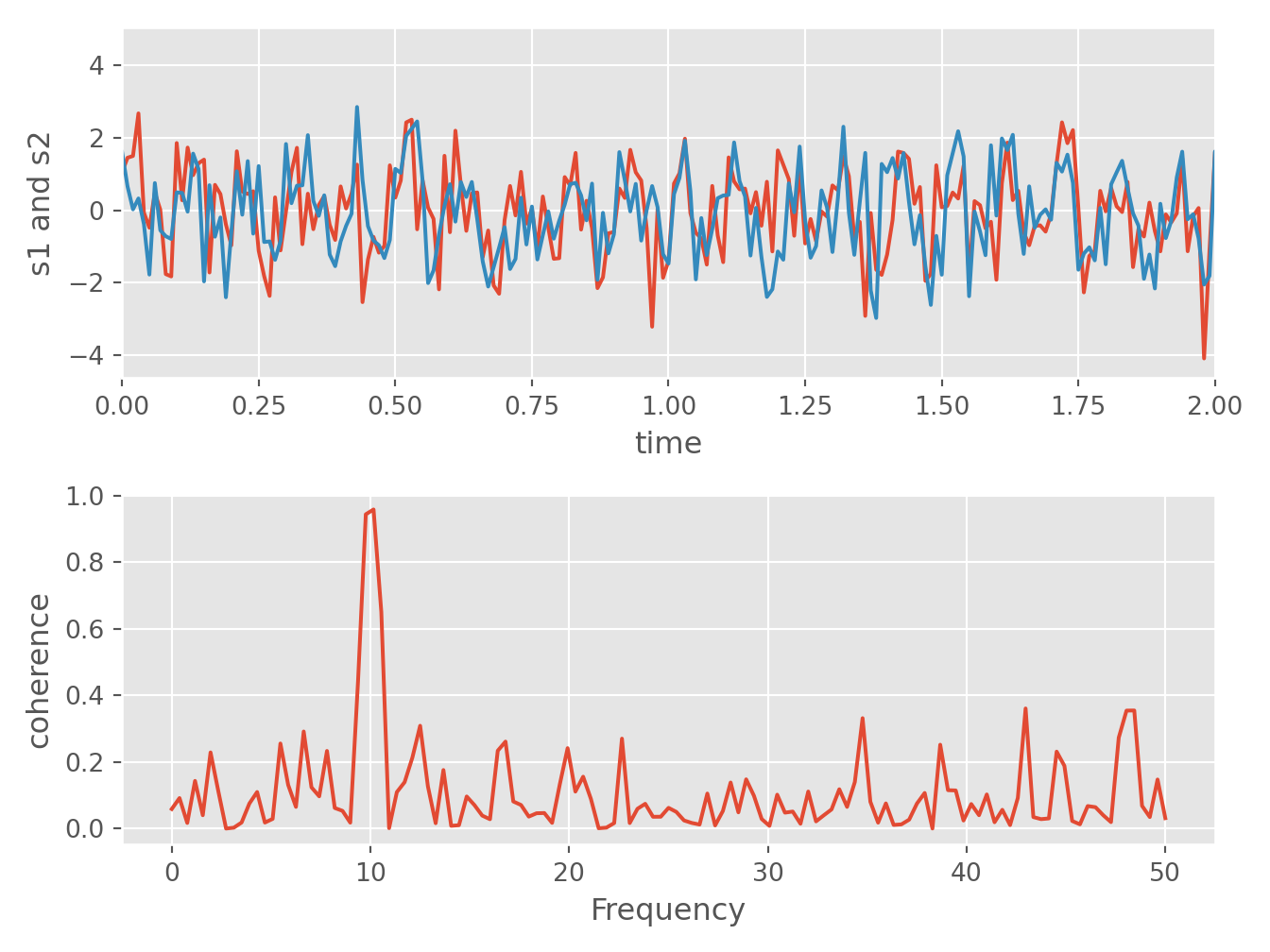
1.5 And also 3D …
Although in data science 3D plots are not recommended, if there is a compelling case where a 3D plot explains a discovery better than a 2D plot, then, it should be okay and justified. But the rule is not abusing of 3D. What you are trying to convey is information per square centimeter of graphics.
Python
# https://matplotlib.org/2.0.2/examples/mplot3d/contour3d_demo.html
from mpl_toolkits.mplot3d import axes3d
import matplotlib.pyplot as plt
from matplotlib import cm
= plt.figure()
fig = fig.add_subplot(111, projection='3d')
ax = axes3d.get_test_data(0.05)
X, Y, Z = ax.contour(X, Y, Z, cmap=cm.coolwarm)
cset =9, inline=1)
ax.clabel(cset, fontsize
plt.show()
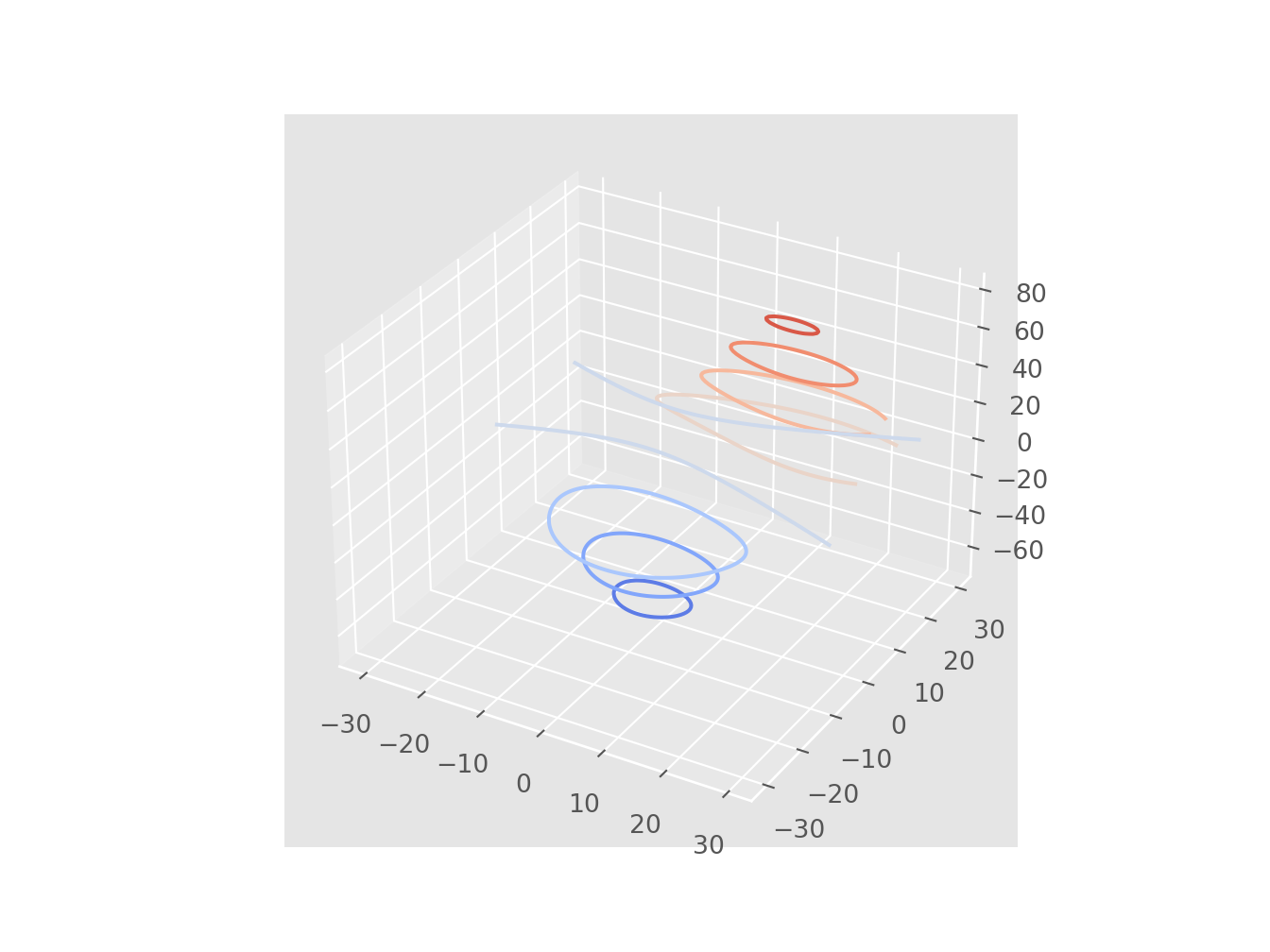